Kernel based methods exhibit well-documented performance in various nonlinear learning tasks. Most of them rely on a preselected kernel, whose prudent choice presumes task-specific prior information. Especially when the latter is not available, multi-kernel learning has gained popularity thanks to its flexibility in choosing kernels from a prescribed kernel dictionary. Leveraging the random feature approximation, this talk will introduce first for static setups a scalable multi-kernel learning approach (termed Raker) to obtain the sought nonlinear learning function ‘on the fly,’ bypassing the `curse of dimensionality’ associated with kernel methods. We will also present an adaptive multi-kernel learning scheme (termed AdaRaker) that relies on weighted combinations of advices from hierarchical ensembles of experts to boost performance in dynamic environments. The weights account not only for each kernel’s contribution to the learning process, but also for the unknown dynamics. Performance is analyzed in terms of both static and dynamic regrets. AdaRaker is uniquely capable of tracking nonlinear learning functions in environments with unknown dynamics, with analytic performance guarantees. The approach is further tailored for online graph-adaptive learning with scalability and privacy. Tests with synthetic and real datasets will showcase the effectiveness of the novel algorithms.
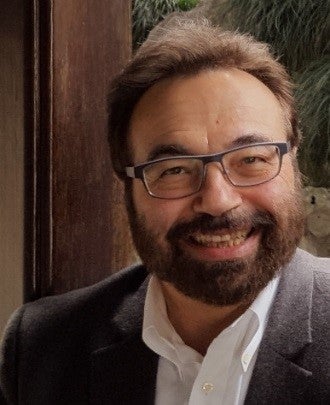
Georgios B. Giannakis (Fellow’97) received his Diploma in Electrical Engr. (EE) from the Ntl. Tech. Univ. of Athens, Greece, 1981. From 1982 to 1986 he was with the U. of Southern California (USC), where he received his MSc. in EE, 1983, MSc. in Mathematics, 1986, and Ph.D. in EE, 1986. He was with the U. of Virginia from 1987 to 1998, and since 1999 he has been with the U. of Minnesota, where he holds a Chair in Wireless Communications, a U. of Minnesota McKnight Presidential Chair in ECE, and serves as director of the Digital Technology Center. His general interests span the areas of statistical learning, communications, and networking - subjects on which he has published more than 460 journal papers, 760 conference papers, 26 book chapters, two edited books and two research monographs. Current research focuses on Data Science with applications to brain, and power networks with renewables. He is the (co-) inventor of 33 patents issued, and the (co-) recipient of 9 best journal paper awards from the IEEE Signal Processing (SP) and Communications Societies, including the G. Marconi Prize Paper Award in Wireless Communications. He also received the IEEE-SPS Nobert Wiener Society Award (2019); Technical Achievement Awards from the SP Society (2000) and from EURASIP (2005); the IEEE ComSoc Education Award (2019); the G. W. Taylor Award for Distinguished Research from the University of Minnesota, and the IEEE Fourier Technical Field Award (inaugural recipient in 2015). He is a Fellow of the National Academy of Inventors, IEEE and EURASIP, and has served the IEEE in a number of posts, including that of a Distinguished Lecturer for the IEEE-SPS.