Learning has shown great success in recent years in controlling complex dynamical systems mainly in the virtual domain. However, this success has not always been matched in the physical world. One of the reasons being that physical systems are required to satisfy a set of operation constraints, such as safety or communication constraints. Designing a single reward that represents all the (possibly) conflicting requirements is a challenging task. I propose instead, to express these requirements as constraints thus formulating a constrained reinforcement learning problem. In this talk, I will establish that solving these problems does not impose an extra computational burden as compared to solving classic reinforcement learning problems.
Having discussed a systematic approach to how to learn policies that satisfy a set of constraints, in the second part of the talk I will tackle the fundamental problem of which constraints should be satisfied in the first place. This decision making aspect of autonomy is both fundamental and challenging, especially when agents must make decisions that violate their specifications. This is critical when multiple tasks and constraints are simultaneously required from the agent, resulting in infeasible settings. These situations arise due to over-specification, scenario uncertainty, or changing operating conditions, and are only aggravated when dynamical system models are learned through simulations.
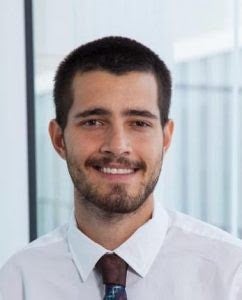
Santiago Paternain received the B.Sc. degree in electrical engineering from Universidad de la República Oriental del Uruguay, Montevideo, Uruguay in 2012, the M.Sc. in Statistics from the Wharton School in 2018 and the Ph.D. in Electrical and Systems Engineering from the Department of Electrical and Systems Engineering, the University of Pennsylvania in 2018. He was the recipient of the 2017 CDC Best Student Paper Award and the 2019 Joseph and Rosaline Wolfe Best Doctoral Dissertation Award from the Electrical and Systems Engineering Department at the University of Pennsylvania. His research interests include optimization and control of dynamical systems.