Reinforcement learning (RL) has achieved tremendous success in solving sequential decision making problems, typically with the aim of finding a policy that maximizes an expected total reward. In practice, it is often critical to further ensure the satisfaction of various safety constraints (for example, a robot working in a warehouse should not hit the arm on the shelf), which thus motivates an emerging field of safe RL. Despite the empirical success, existing safe RL algorithms do not achieve the best possible convergence rate, and often fail to converge to the globally optimal policy.
In this talk, I will present our recent studies that provably improve the existing two major safe RL approaches. I will first present a new primal-dual safe RL algorithm and show that such an algorithm attains a globally optimal policy and improves the best known computational complexity of the existing algorithms. I will then present another new primal-type safe RL algorithm and further provide the characterization of its global optimality guarantee and convergence rate. Our experiments demonstrate that the primal safe RL algorithm substantially outperforms the primal-dual algorithm. I will conclude my talk with further intuition about the comparison between these two approaches and future directions on the topic.
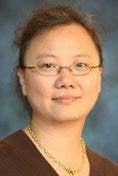
Dr. Yingbin Liang is currently a Professor at the Department of Electrical and Computer Engineering at the Ohio State University (OSU). She received the Ph.D. degree in Electrical Engineering from the University of Illinois at Urbana-Champaign in 2005, and served on the faculty of University of Hawaii and Syracuse University before she joined OSU. Dr. Liang's research interests include machine learning, optimization, information theory and statistical signal processing. Dr. Liang received the National Science Foundation CAREER Award and the State of Hawaii Governor Innovation Award in 2009. She also received EURASIP Best Paper Award in 2014. She served as an Associate Editor for the Shannon Theory of the IEEE Transactions on Information Theory during 2013-2015.