Mobile and embedded computing devices have become key carriers of deep learning to facilitate the widespread use of machine intelligence. However, there is a widely recognized challenge to achieve real-time DNN inference on edge devices, due to the limited computation/storage resources on such devices. Model compression of DNNs, including weight pruning and weight quantization, has been investigated to overcome this challenge. However, current work on DNN compression suffers from the limitation that accuracy and hardware performance are somewhat conflicting goals difficult to satisfy simultaneously.
We present our recent work CoCoPIE, representing Compression-Compilation Codesign, to overcome this limitation towards the best possible DNN acceleration on edge devices. We propose novel fine-grained structured pruning schemes, including pattern-based pruning, block-based pruning, etc. They can simultaneously achieve high hardware performance (similar to filter/channel pruning) while maintaining zero accuracy loss, with the help of a compiler, which is beyond the capability of prior work. Similarly, we present a novel quantization scheme that achieves ultra-high hardware performance close to 2-bit weight quantization, with almost no accuracy loss. Through the CoCoPIE framework, we are able to achieve real-time on-device execution of a number of DNN tasks, including object detection, pose estimation, activity detection, speech recognition, just using an off-the-shelf mobile device, with up to 180 X speedup compared with prior work. Our comprehensive demonstrations are at : https://www.youtube.com/channel/UCCKVDtg2eheRTEuqIJ5cD8A. Last we will introduce our recent breakthrough of superconducting logic based neural network acceleration that achieves 10^6 times energy efficiency gain compared with state-of-the-art solutions, achieving the quantum limit in computing.
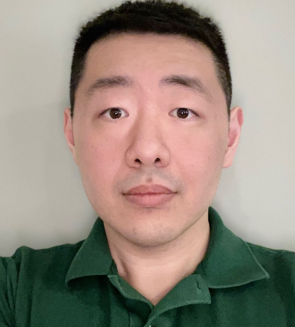
Yanzhi Wang is currently an associate professor and faculty fellow at Dept. of ECE at Northeastern University, Boston, MA. He received the B.S. degree from Tsinghua University in 2009, and Ph.D. degree from University of Southern California in 2014. His research interests focus on model compression and platform-specific acceleration of deep learning applications. His work has been published broadly in top conference and journal venues (e.g., DAC, ICCAD, ASPLOS, ISCA, MICRO, HPCA, PLDI, ICS, PACT, ISSCC, AAAI, ICML, NeurIPS, CVPR, ICLR, IJCAI, ECCV, ICDM, ACM MM, FPGA, LCTES, CCS, VLDB, PACT, ICDCS, RTAS, Infocom, C-ACM, JSSC, TComputer, TCAS-I, TCAD, TCAS-I, JSAC, TNNLS, etc.), and has been cited above 13,600 times. He has received six Best Paper and Top Paper Awards, and one Communications of the ACM cover featured article. He has another 13 Best Paper Nominations and four Popular Paper Awards. He has received the U.S. Army Young Investigator Program Award (YIP), IEEE TC-SDM Early Career Award, APSIPA Distinguished Leader Award, Massachusetts Acorn Innovation Award, Martin Essigmann Excellence in Teaching Award, Massachusetts Acorn Innovation Award, Ming Hsieh Scholar Award, and other research awards from Google, MathWorks, etc. He has received 25 federal grants from NSF, DARPA, IARPA, ARO, ARFL/AFOSR, Dept. of Homeland Security, etc.. He has participated in a total of $40M funds with personal share $8.5M. Six of his former Ph.D./postdoc students become tenure track faculty at Univ. of Connecticut, Clemson University, Chongqing University, Beijing University of Technology, Texas A&M University, Corpus Christi, and Cleveland State University. They have secured around $3.5M personal share in funds.