The increasingly more complex artificial intelligence (AI) models have attracted interdisciplinary efforts to speed up AI workloads. On the hardware front, the disruptive paradigm of compute-in-memory (CIM) aims to process data directly inside memory arrays, which is a promising approach to overcome the memory wall problem that conventional platforms face. Complex arithmetic operations can be performed using compute-capable CIM arrays to achieve massive parallelism and high energy efficiency. This talk focuses on the circuit design and hardware architecture aspects of CIM for executing AI inference. First, I will present a mixed-signal CIM engine that performs parallelized matrix multiplications inside resistive random-access memory (RRAM) arrays. The RRAM-based CIM chip, fabricated in TSMC 40-nm node, demonstrates circuit techniques that ensure PVT-robust computing. Next, I will discuss the unique opportunities to transform computing inside heterogeneous 3D stacks. An 3D-stacked CIM accelerator targeting vision transformer models is shown to gain form factor and efficiency benefits. Finally, I will present a low-power portable ultrasound system to showcase how CIM can be applied to embedded electronics. Through data volume reduction of the ultrasound frontend and the efficient on-device intelligence provided by CIM, significant power savings can be achieved for the portable imaging system.
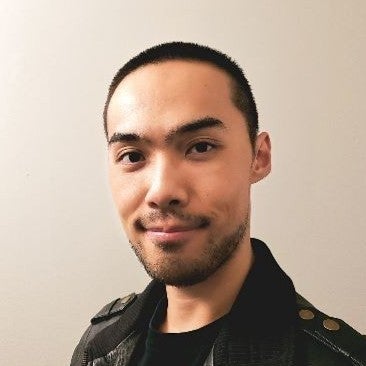
Wantong Li is a Ph.D. candidate in electrical and computer engineering at the Georgia Institute of Technology, advised by Dr. Shimeng Yu. His research centers on memory-centric computing platforms, spanning areas of efficient and robust IC design, heterogeneous 3-D integrated systems, and domain-specific architecture. He received a BSEE degree from Washington University in St. Louis in 2015 and a MSEE degree from Columbia University in 2016. From 2017 to 2019, he worked as an IC Design Engineer at Power Integrations. He also held internship positions at AMD, MediaTek, and Roche Diagnostics. He was the recipient of the Columbia University Nikola Tesla Scholarship and the Georgia Tech ECE INSPIRE Fellowship.