With the advent in computational infrastructure and smart devices, there has been an exponential growth in the amount of data collected and stored locally on individual devices. Applying machine learning to extract value from such massive data and provide data-driven models and decision making has been a popular research topic. However, porting these large amounts of data to a centralized processing unit for machine learning will face a number of issues, such as communication challenges associated with data transfer and potential privacy issues associated with sharing raw data. In this talk I will present our recent research on decentralized Bayesian learning algorithms that allow networked agents to collaboratively reconstruct global posterior of unknown model parameters from distributed data sets. The developed decentralized algorithms only require the agents to interact with their neighboring agents to learn a model that has the same accuracy as a centrally trained model.
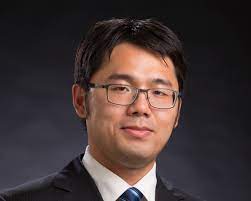
Dr. He Bai received his B.S. degree from the University of Science and Technology of China in 2005, and M.S. and Ph.D. degrees in electrical engineering from Rensselaer Polytechnic Institute, Troy, NY, in 2007 and 2009, respectively. From 2009 to 2010, he was a Postdoctoral Researcher in Mechanical Engineering with Northwestern University, Evanston, IL. From 2010 to 2015, he was a Senior Research and Development Scientist with UtopiaCompression Corporation, where he managed a number of R&D projects on unmanned aerial systems detect-and-avoid, target tracking, and target handoff in GPS-denied environments. In 2015, he joined Oklahoma State University, where he is currently an associate professor in mechanical and aerospace engineering. His research interests include decentralized learning, nonlinear control and estimation, robotics, cooperative driving, and multi-agent systems.