Artificial intelligence (AI) models that have billions of parameters exacerbate poor energy efficiency on conventional general-purpose processors such as GPUs and CPUs. Analog in-memory computing, or simply analog AI, is a promising approach to addressing the challenge, which is predicted to enjoy energy efficiencies 40-140 times higher than those of cutting-edge GPUs. However, training AI models on analog devices is difficult and largely unexplored. Recent empirical studies have shown that the "workhorse" of AI training - stochastic gradient descent (SGD) algorithm performs poorly when applied to train models on non-ideal analog devices.
In this talk, we will propose a mathematical model to accurately characterize training dynamics on analog devices. Building upon this, for the first time, we will uncover the role of underlying device physics on the training dynamics, and then demystify the non-convergence issues of vanilla SGD-based training on non-ideal analog devices that have asymmetric updates, reading noise, and device variability. We will further discuss how to algorithmically mitigate the asymmetric error induced by training on non-ideal analog devices and hence eliminate the asymptotic training error. We will conclude the talk by presenting some simulations that verify the correctness of the theoretical analyses and pointing out future directions in this promising area.
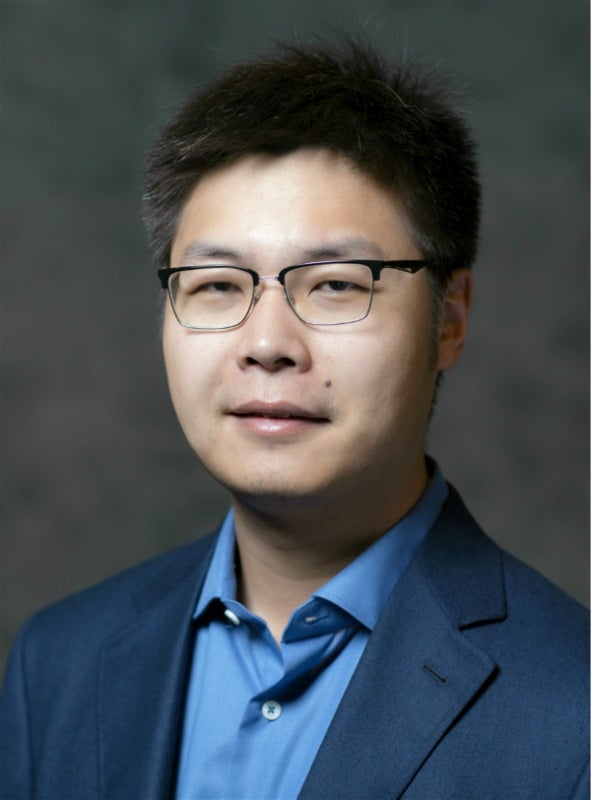
Tianyi Chen (https://sites.ecse.rpi.edu/~chent18/) is an Assistant Professor in the Department of Electrical, Computer, and Systems Engineering at Rensselaer Polytechnic Institute (RPI), where he is jointly supported by the RPI - IBM Artificial Intelligence Research Partnership. Dr. Chen received his B. Eng. degree from Fudan University in 2014, and the Ph.D. degree from the University of Minnesota in 2019. Dr. Chen's research centers on the theoretical foundations of optimization and machine learning, with a focus on their applications to emerging data processing and computing paradigms. Dr. Chen is the inaugural recipient of IEEE Signal Processing Society Best PhD Dissertation Award in 2020, a recipient of NSF CAREER Award in 2021, and faculty research awards from Amazon, Cisco and IBM. He is also the co-author of several best paper awards including one at IEEE International Conference on Acoustics, Speech and Signal Processing (ICASSP) in 2021.