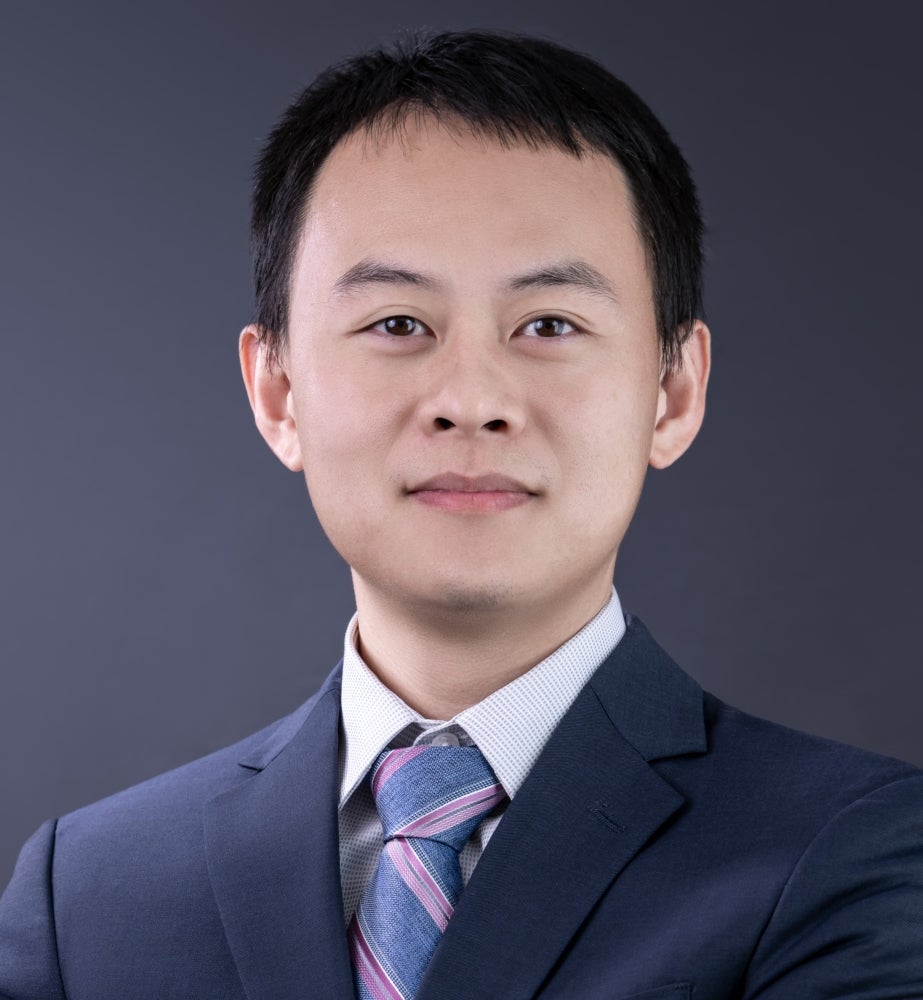
ECSE Assistant Professor Dr. Liu Liu has received an NSF CAREER award from the Division of Computing and Communication Foundations (CCF) for his project, “CAREER: Elastic Algorithm-Architecture Co-Design for Energy-Efficient and Scalable AI via Dynamic Connectivity.”
Dr. Liu’s research aims to reduce AI’s rapidly growing energy footprint by rethinking how AI hardware processes, stores, and transfers data. Through a novel “elastic” approach that enables AI models and architectures to adapt dynamically, Dr. Liu and his team will tackle the challenge of maintaining high performance while cutting redundant computations. The project will include education and outreach activities that engage students and foster diversity in computing, thus broadening participation in driving more sustainable and scalable AI advancements.
Dr. Liu joined ECSE in 2022 after obtaining his Ph.D. degree from the Computer Science Department at the University of California Santa Barbara. Liu received his M.S. in Computer Engineering in 2015, also from UC Santa Barbara, and his B.Eng. in Information Display and Optoelectronics from the University of Electronic Science and Technology in China. Liu’s research interest lies in the intersection of computer architecture and AI/ML. He focuses on the hardware/algorithm co-design for AI/ML systems to enhance efficiency, performance, and robustness. A major bottleneck to performance improvement in deep learning is the exponential increase in computation cost. Liu’s work directly addresses this issue by exploiting the “elasticity” in AI/ML computational architectures, which means architectural flexibility in terms of computational resources and data movement without severely impacting performance.