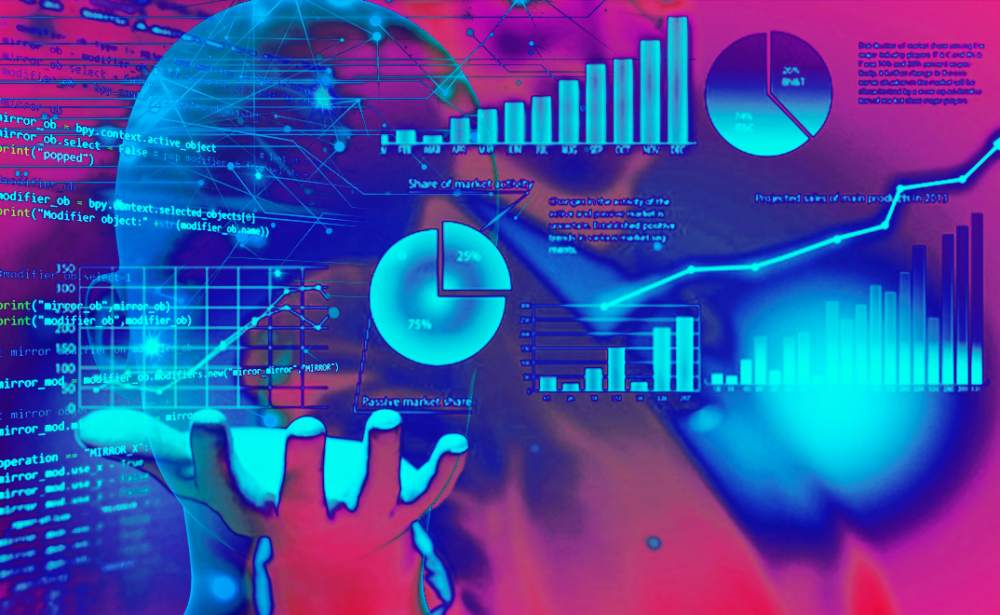
Professor Wang's proposal is centered on data processing and information extraction from high-dimensional datasets obtained by Air Force networks and systems. The large amounts of data consume a lot of communication bandwidth and place an overwhelming requirement on the Air Force workforce for data processing. The goal of this project is to develop computationally efficient and cyber-resilient methods for data recovery, anomaly identification, and information extraction with provable guarantees. Central to this proposal is to exploit instinct low-dimensional structures in the data and develop new non- parametric models to characterize these structures. The expected research outcomes include a class of data recovery, data clustering, and machine learning methods based on these models, together with the algorithmic designs, theoretical analyses, and numerical evaluations. These approaches can be implemented in real time, apply to diverse datasets, and are robust to cyber data attacks. Different from conventional data-driven and model-based methods, this work has a unique angle of developing model-motivated data-orientated methods for information extraction from large datasets. This proposal will extend the theory of low-dimensional-model-based data analyses by developing new models that further characterize the temporal correlations in the data. It broadens the scope of nonconvex-optimization-based learning approaches by developing new computationally efficient algorithms with provable performance guarantees. By connecting deep learning with low-dimensional models, it simplifies the neural network architecture and develops fast learning algorithms that are guaranteed to find appropriate filter parameters for satisfactory testing performance. The research efforts will enhance information extraction from networked data and the resilience to cyber data attacks in Air Force systems.