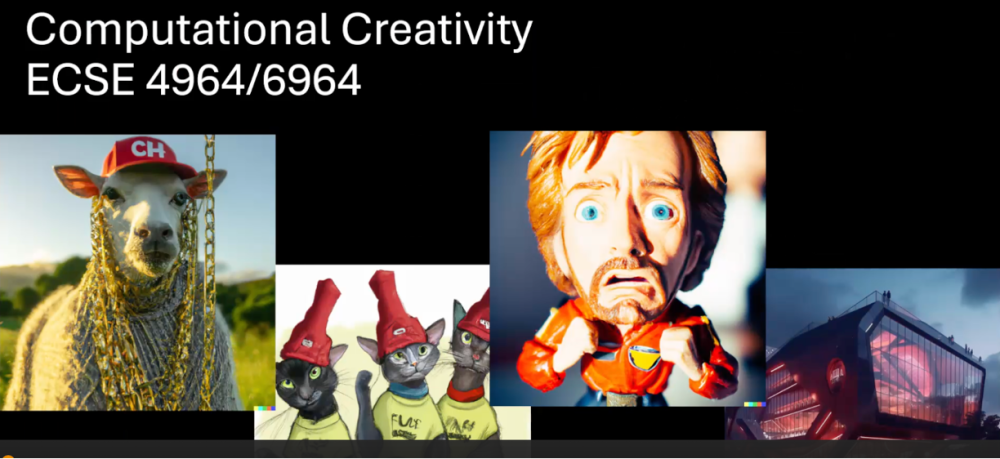
Rich Radke offered a unique course on computational creativity in fall 2023, covering the fast moving field of generative AI. He described below his journey through this course including his motivation, approach, experience, and results of the course.
OpenAI’s release of DALL-E 2 in the spring of 2022 was the first time I really took notice of how incredible generative models for image creation had become. As a computer vision grad student and professor, I was familiar with the “old school” of neural networks (backpropagation, hidden Markov models) but the images my friends and I were producing in a matter of seconds seemed like an impossible extension, almost in the realm of science fiction. In early 2023, I bit the bullet and requested to teach a new special topics class called Computational Creativity in the fall semester, covering the last ten years of rapidly evolving research in generative image and text synthesis, with the ulterior motive of getting myself up to speed! I also wanted to explore how artists have incorporated generative models in their work, and discuss the intersections of technology with law, ethics, and philosophy. I lined up a couple great guest speakers: Aaron Hertzmann from Adobe Research, discussing the question of whether computers can be considered artists, and Pamela Samuelson from UC Berkeley discussing legal challenges to generative AI.
The pilot offering of the class just wrapped up in Fall 2023, with an enthusiastic group of about 20 seniors and grad students. You can follow along at the course web page here; while some files are available only to members of the RPI community, all the lectures are publicly available in this YouTube playlist. We started with the by-now classical variational autoencoder or VAE (even though it was only introduced about 10 years ago!) and proceeded through the many kinds of generative adversarial networks (GANs), diffusion models, transformers, and large language models. We also overviewed generative models for creating music, 3D objects, vector graphics, and animations. In many lectures, we were discussing innovations that had just been announced a few months ago, and in fact, it became impossible to keep up with the impressive new tools revealed over the course of the fall (e.g., OpenAI’s DALL-E 3, Stability AI’s Stable Video Diffusion, and Meta’s AudioCraft).
At the end of the semester I edited some of the best student work of the semester into my traditional final video. The students worked in teams on mini-projects over the course of the semester, collecting their own training data and building generative models to create similar inputs. Every assignment involved a peer evaluation so that students could view and comment on each others’ work. The students’ open-ended final projects included creating stylized environments to better train agents for reinforcement learning; creating lyrics, music, visuals and album covers for music videos; telling 5-panel stories with emotional beats; and playing a Pictionary-type game with a human and AI taking turns drawing and guessing. I especially liked this project that renders familiar parts of campus in a dreamy anime style, and this project that creates short narrated video stories from an initial user prompt.
Teaching this course was like being a graduate student again; I reviewed hundreds of papers and learned a whole new area. It was also exhausting; I’m looking forward to teaching a couple of courses where the state of the art doesn’t change every few weeks!