We deal with the combinatorial problem of learning directed acyclic graph (DAG) structure from observational data adhering to a linear structural equation model (SEM). Leveraging advances in differentiable, nonconvex characterizations of acyclicity, recent efforts have advocated a continuous constrained optimization paradigm to efficiently explore the space of DAGs. Most existing methods employ lasso-type score functions to guide this search, which (i) require expensive penalty parameter retuning when the SEM noise variances change across problem instances; and (ii) implicitly rely on limiting homoscedasticity assumptions. In this talk, I will propose a new convex score function for sparsity-aware learning of linear DAGs, which incorporates concomitant estimation of scale and thus effectively decouples the sparsity parameter from noise levels. Regularization via a smooth, nonconvex acyclicity penalty term yields CoLiDE (Concomitant Linear DAG Estimation), a regression-based criterion amenable to efficient gradient computation and closed-form estimation of exogenous noise levels in heteroscedastic scenarios. The algorithm outperforms state-of-the-art methods without incurring added complexity, especially when the DAGs are larger, and the noise level profile is heterogeneous. CoLiDE exhibits enhanced stability manifested via reduced standard deviations in several domain-specific metrics, underscoring the robustness of the novel linear DAG estimator.
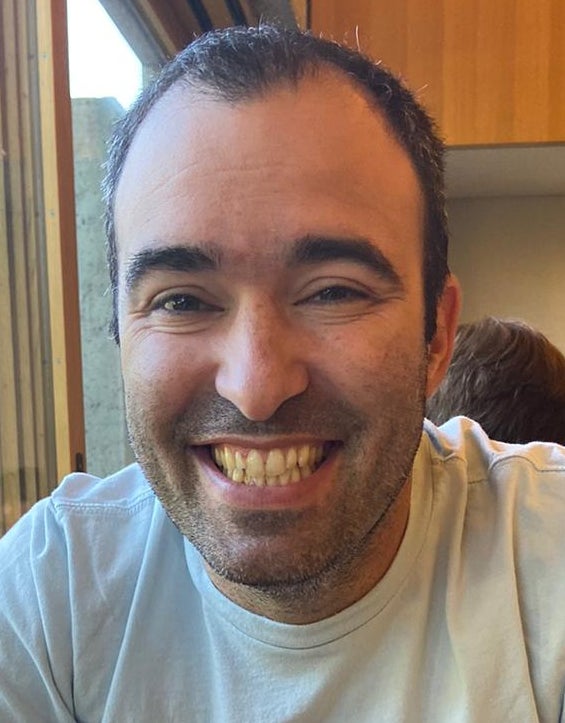
Gonzalo Mateos earned the B.Sc. degree from Universidad de la Republica, Uruguay, in 2005, and the M.Sc. and Ph.D. degrees from the University of Minnesota, Twin Cities, in 2009 and 2011, all in electrical engineering. He joined the University of Rochester, Rochester, NY, in 2014, where he is currently an Associate Professor with the Department of Electrical and Computer Engineering, the Department of Computer Science (secondary appointment), as well as the Associate Director for Research at the University of Rochester's Goergen Institute for Data Science. He also was the Asaro Biggar Family Fellow in Data Science (2020-23). During the 2013 academic year, he was a visiting scholar with the Computer Science Department at Carnegie Mellon University. From 2004 to 2006, he worked as a Systems Engineer at Asea Brown Boveri (ABB), Uruguay. His research interests lie in the areas of statistical learning from complex data, network science, decentralized optimization, and graph signal processing, with applications in brain connectivity, causal discovery, wireless network monitoring, power grid analytics, and information diffusion.